AI-powered solutions to improve forecasts
It's definitely challenging to keep up with all the AI news these days, but there's yet another area where AI breakthroughs are bringing significant changes to the business world, and that's in forecasting.
Demand forecasting refers to predicting future demand for a product or service. Predicting demand is crucial to optimize supply chains, inventory management, staffing, and marketing activities. An increasing number of businesses are now applying machine learning to forecasting for more flexible and dynamic forecasting tools. As a result, forecasts are becoming more long-term and precise, yielding accurate insights for strategic decision-making.
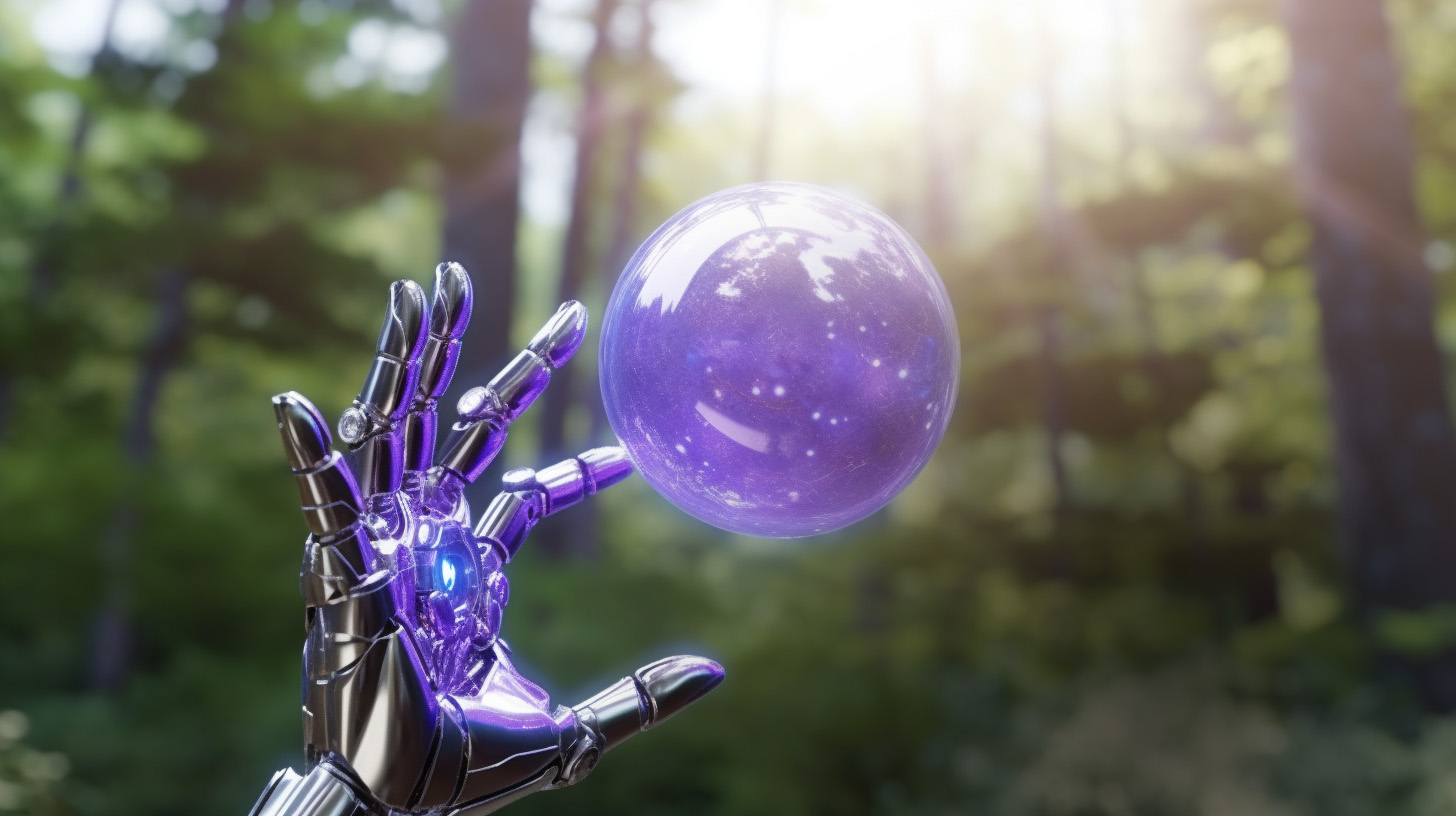
What is forecasting?
The forecasting field involves making predictions about the future based on historical and current data. Demand forecasting, in turn, aims to estimate future demand for a specific product or service. A reliable forecast allow businesses to correctly anticipate the quantity of certain goods that will be demanded at a particular place and time.
Forecasting future demand for products is not a new concept for businesses. Traditionally, the field has been dominated by statistical methods (such as Exponential Smoothing, ARMA) that use historical sales figures to estimate future demand, which can still provide good forecasts. However, in many cases, demand will be influenced by external factors that are difficult to incorporate into traditional statistical models. This can create misleading forecasts with a high margin of error.
AI-driven forecasts
Using machine learning in forecasting is not a new concept, but significant breakthroughs in areas like natural language processing (e.g., Transformer models) are now making their way into the domain. By now, you're probably familiar with OpenAI's ChatGPT, and the "T" in GPT stands for "Transformer." Increased availability of computing power is another reason why AI solutions in forecasting have truly started to gain momentum.
An article by McKinsey highlights significant benefits of the technology - AI-driven forecasting throughout the supply chain can reduce errors with 20-50%, and lost sales due to products not being available can be reduced by up to 65%.
"AI-driven forecasting essentially addresses the same issues as the classical, traditional methods, but applying AI means that we can modify and optimize the technology to fit the purpose better. Increased understanding and higher precision are the major differences compared to traditional forecasting methods," says Johannes Koch, forecasting specialist and partner at Violet AI.
By leveraging machine learning in forecasts, you can go beyond identifying recurring patterns related to for example weekdays, holidays, and seasons. This approach allows for integrating numerous factors that may influence demand, such as:
- Internal factors, for instance, can have an impact – such as demand increasing during price reductions or promotional campaigns. Moreover, product visibility, like securing a prominent store or website placement, can also drive demand.
- External factors, in addition to internal ones, play a role as well. Weather conditions, competitor pricing changes, and local events such as concerts or football matches near physical stores can all have an influence on customer demand.
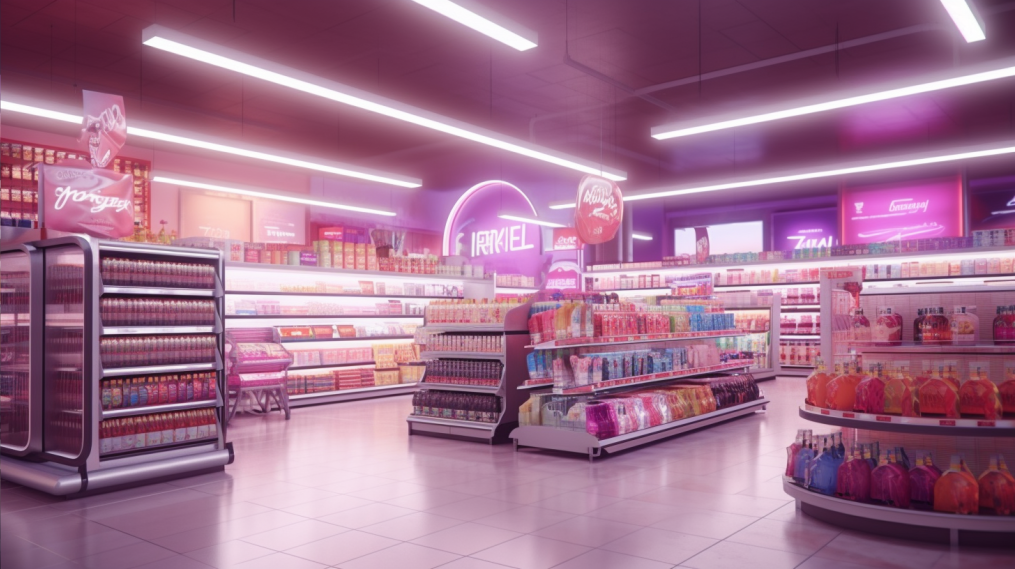
Improved forecasts to reduce food waste
One example where AI-driven forecasting can be highly valuable is in cases where the sales cycles are very short, such as in the case of fresh food. Ordering too much products results in unnecessary waste, while ordering too little risks missed sales opportunities. Violet is helping a company in the food industry in forecasting demand for their products across 1000+ stores. The AI solution takes sales data from different stores in account and learns from common patterns like weekly profiles and the influence of weather conditions.
Mikael Lindstedt Nelsson, Data Scientist, is one of the experts from Violet working on the project:
“This particular company is well ahead in its AI maturity. They already had good track of their data flows and good data quality. Forecasting demand is a core part of their deal, and they saw great business value in better and more dynamic forecasting, and more flexible tools. In this project, we have really tested the limits of what AI-driven forecasting can accomplish,” says Mikael.
Enhanced understanding of demand
Businesses that tie up substantial capital in their products can achieve significant gains when applying AI to their forecasts. Even slight improvements in predictions can unlock substantial amounts of capital. Violet is currently partnering with a fuel company to tackle this challenge. The company strives to meet the demand at their depots across Sweden while avoiding unnecessary capital allocation. Traditional forecasting methods have proven inadequate in accurately estimating the right volume for a given time period. In response, Karin Johansson and Johannes Koch from Violet has taken on the task of elevating the forecasts to new heights with machine learning.
Karin Johansson, Machine Learning Engineer, shares insights into the technology they are currently developing:
"We have built models that forecast 45 days ahead, providing a clearer understanding of demand. The solution is a state-of-the-art model," says Karin.
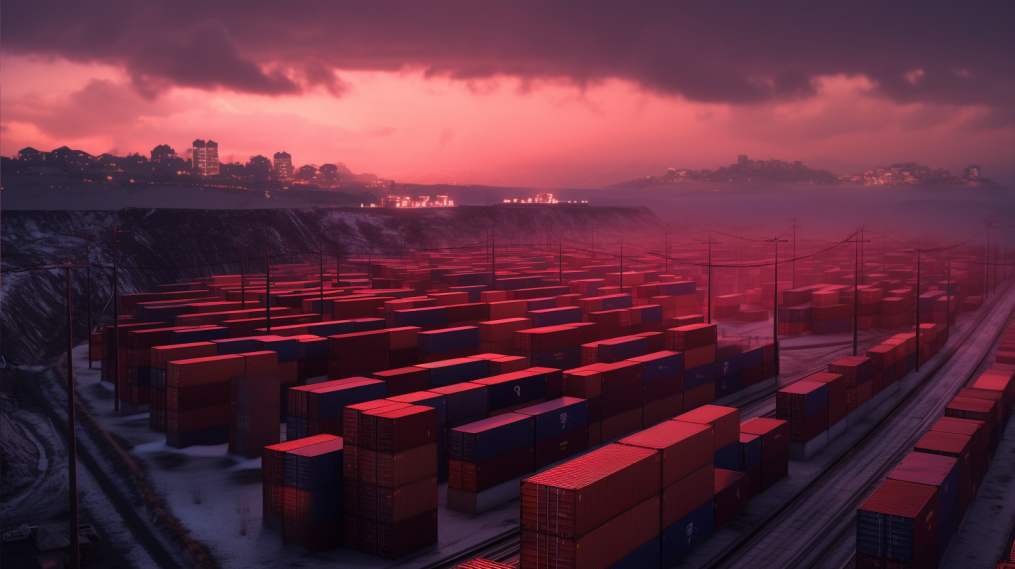
No technology is free from challenges
While the potential of AI is high, there are still challenges in forecasting. There is no guarantee that complex AI techniques will yield better results than simpler, traditional methods. Insufficient data is often the biggest obstacle, but existing infrastructure can also impose limitations on what actually can be accomplished.
"Good or bad data is key. You can have 100 million data points, but if the data is incorrect, it doesn't matter how much data we have or what methods we use. Therefore, part of our job is to clean the data and remove anything that is misleading so that the models are not trained on inaccurate data," says Mikael.
Research to share
Tech giants like Google and Facebook are industry leaders in AI solutions, and the internal research teams often share their research with the public. Both Facebook and Google have developed their own forecasting models, with Facebook introducing Prophet in 2017 and Google introducing Temporal Fusion Transformer in 2019. Building upon the latest research, smaller companies and individual developers can further enhance the technology and create customized solutions, including Violet.
"To be able to build upon the latest research means that AI can bring greater value to more people, faster. Developing the technology is just one part of our overall process. Once we see that our solutions work from a business perspective, we can shift our focus to scaling up and putting them into production. We continue to optimize the models as long as needed," says Karin.
Next step: explainability
In an era where AI tools are becoming increasingly common in businesses as well as in society at large, an article in Vice highlights the importance of being able to explain how the models work and what they base their conclusions on. This is crucial to build trust and understanding of the models and to make so-called black box models more transparent.
"Focus is starting to shift towards traceability and explainability. That is the next big step for building higher trust in forecasting models. Alongside dynamic and reliable data collection, cognitive understanding of the forecasts will be crucial moving forward," concludes Johannes.
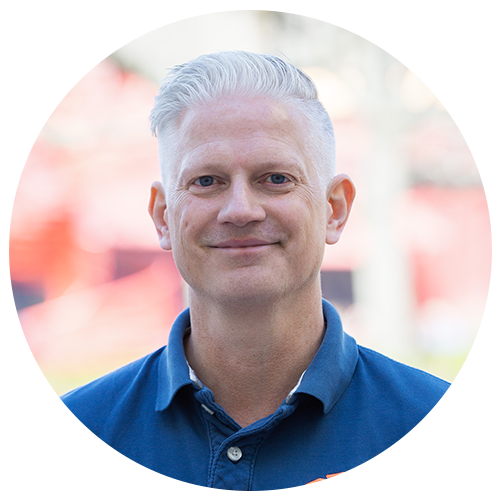
Do you want to take the next step towards smarter forecasting and data-driven decision-making?
Contact me at mattias.guilotte@violet.ai to book a workshop or an inspirational lecture.
About Violet
Violet AI was founded in 2018 as one of the first pure-play AI agencies in the Nordics. Today, Violet consists of a fast-growing consulting and advisory team and four subsidiaries with a total of 50 employees. We specialize in Machine Learning, Advanced Analytics, Intelligent Automation, System Development, and AI Strategy.